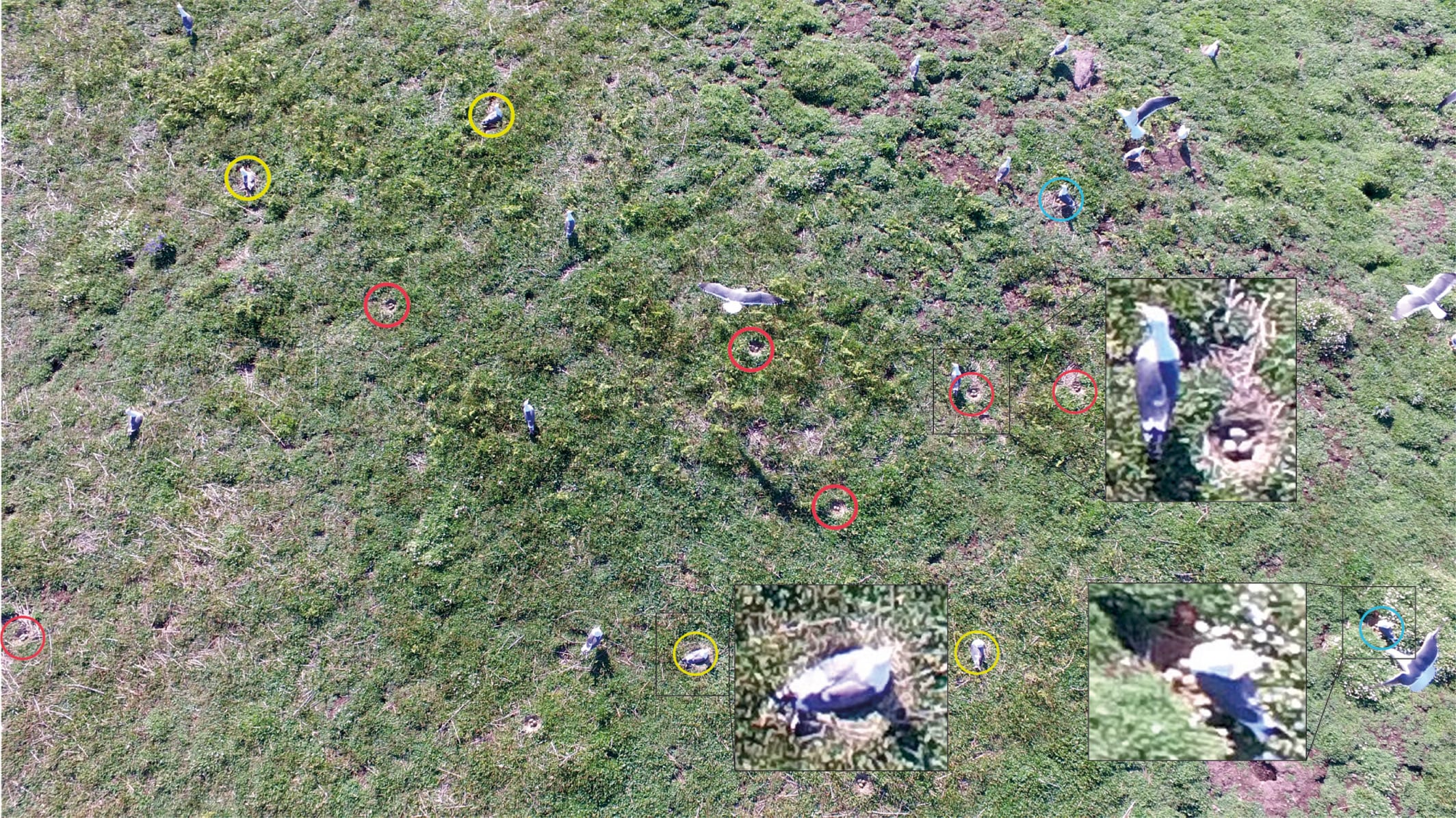
News
Drones for Counting Sea Gulls
Surveying and quantification of wild life in different parts of the world is crucial to our understanding of ecosystem and environment and how it is changing as a function of natural and artificial changes. For this reason, wild life in different habitat is monitored regularly and periodically for accurate quantification. While the procedures and methodologies undertaken for this task are majorly based on approximation and tedious counting, technological advancements and recent innovations highlight tremendous potential that can significantly shorten the time taken by these procedures while being extremely convenient, sustainable and feasible for application.
Keeping a count of sea gulls can be made much more convenient by using UAVs or drones that literally provide a “bird’s eye” view of the site they observe. But while drones and other aerial robots can record sea gulls in different locations, it is still a technical challenge to translate this recorded imagery or data into survey data that can be quantified. Seabirds, in particular, present the particular challenges of nesting in large, often inaccessible colonies that are difficult to view for ground observers, which are commonly susceptible to disturbance.
To counter this problem and to develop a system that practically useful for counting of sea gulls, researchers at the University of York and the University of Gloucestershire in UK formulated a research paper titled, “Can drones count gulls? Minimal disturbance and semiautomated image processing with an unmanned aerial vehicle for colony-nesting seabirds“. In this publication, they proposed a protocol for carrying out UAV surveys of a breeding seabird colony and subsequent image processing to provide a semi-automated classification for counting the number of birds.
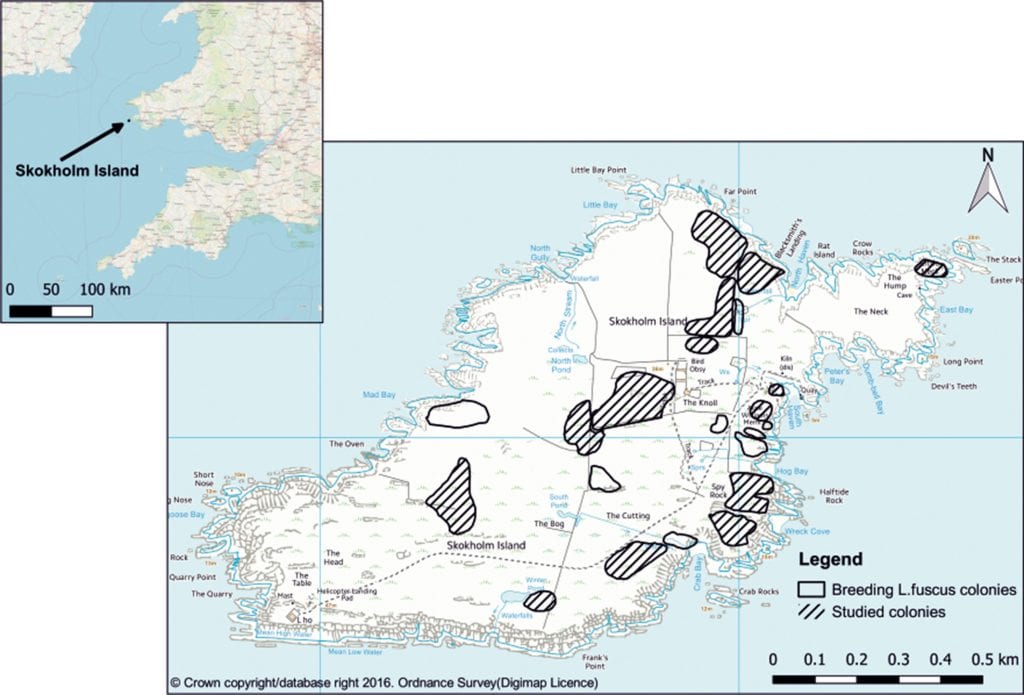
Location map of Skokholm Island and position of Larus fuscus breeding colonies, indicating those that were used in this study
The researchers extensively studied the behavioral patterns of sea gulls and adjusted their algorithm to account for changes in the movements of the birds with time and location. The crucial factor considered when studying behavioral patterns was to ensure physical isolation of the drones or UAVs from the birds during remote monitoring of sea gulls – protecting the birds from injury due to their impact with the robots and preventing loss of equipment due to bird attacks.
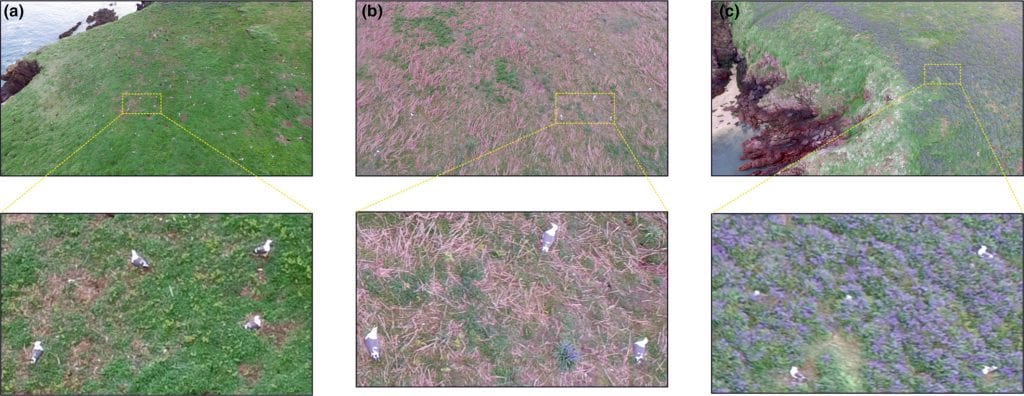
Example imagery captured by the unmanned aerial vehicle flown at 15 m altitude with zoomed areas showing the spatial resolution achieved and Larus fuscus identification on (a) open grassland, (b) rough grass/bracken, Pteridium spp. scrub, and (c) bluebells, Hyacinthoides nonscripta
Behavioral patterns of sea gulls
- Hop— A brief flight of the bird to travel short distances in close locations.
- Flight—A relatively longer flight, hardly around 10 seconds. The sea gull does not approach the UAV or drone during a flight.
- Attack— The researchers tested their drones by flying them over an area full or sea gulls and only one bird attacked one drone for roughly 5 seconds aggressively.
Image processing
Photoscan is a commercially available program that uses algorithms to automatically detect features in the images such as edges and points from the unordered aerial image collection. It is able to convert the images into a single 2D orthomosaic without the individual scale, tilt, and relief distortions of each image.
All images were manually assessed prior to processing and, where necessary, deleted from the subset if they were distorted or blurred from the flying motion. All remaining images for each sub-colony of the bird were added to the software, and image processing followed the recommended procedure.
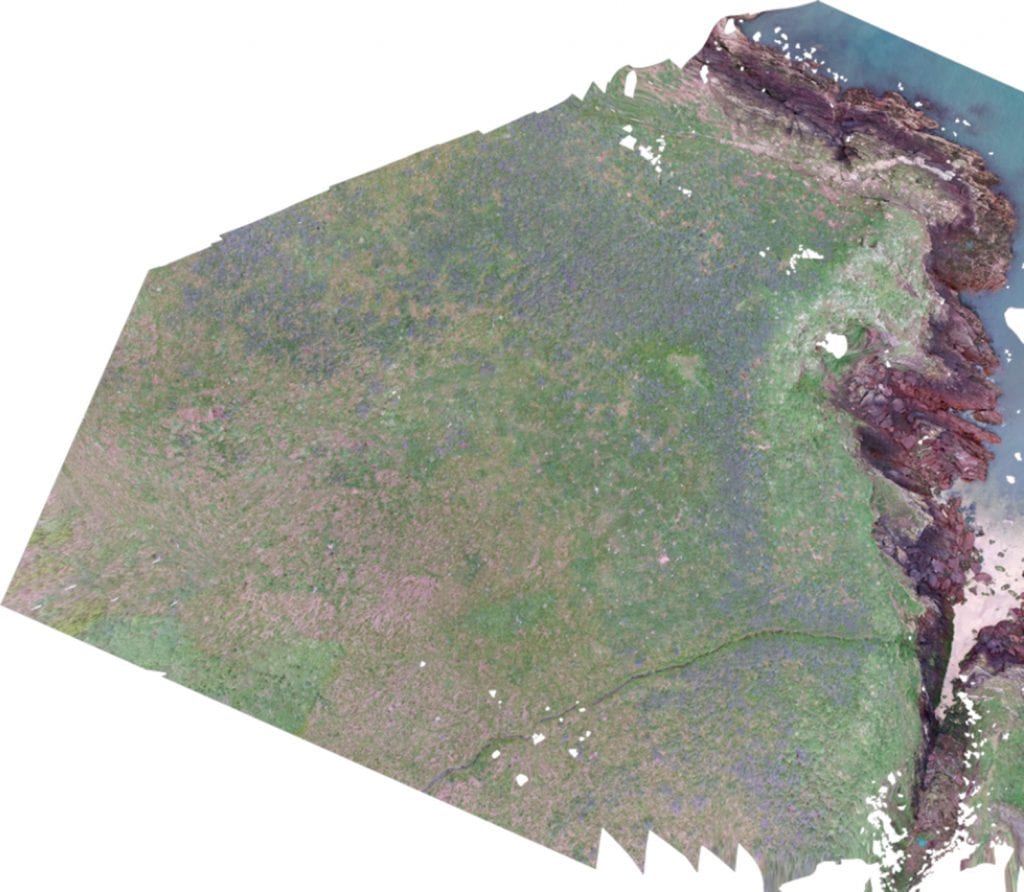
Example of an orthomosaic showing the North Haven subcolony (Colony 3)
Classification
Classification of recorded data acquired by the drones is a user-driven process that involves acquiring a sample of pixels from a known class from the image that provides an accurate representation of the class to create a unique spectral signature for each class; the classification process then automatically separates the image into these.
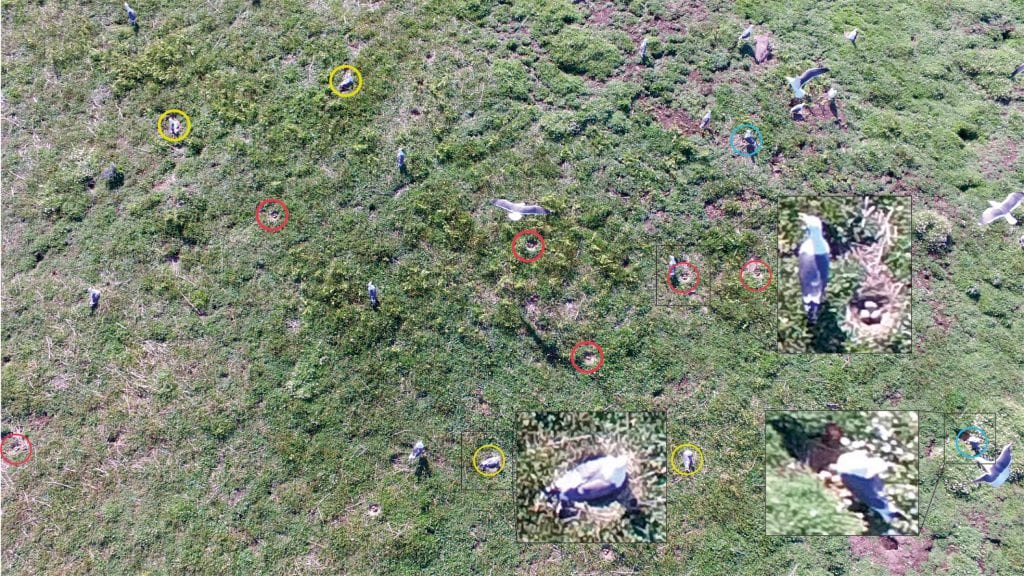
An example of an image captured at 5 m.a.s.e.: Red circles = nests that were temporarily abandoned with eggs clearly visible, blue circles = nests temporarily abandoned with nests and eggs partially visible, yellow circles = nests with apparently incubating adults. Insets show examples of close‐ups of individual birds
Results
The researchers tested their UAVs with their pre-programmed controllers to access 12 different colonies of sea gulls. They made note of some important points,
- Loss of bird nests was not noted in any of the sub colonies studied due to predator attacks or cannibalism.
- There was only instance in which a bird tried to attack a drone flying at an altitude of 15 meters above the ground.
- While there was no impact between a sea gull and a drone save for the attack on the drone mentioned, other bird species like crows did approach drones during their first flight. This was not however a repeated phenomena as other birds stopped approaching UAVs after the first flight.
Citation: Rush GP, Clarke LE, Stone M, Wood MJ. Can drones count gulls? Minimal disturbance and semiautomated image processing with an unmanned aerial vehicle for colony‐nesting seabirds. Ecol Evol. 2018;00:1–13. https://doi.org/10.1002/ece3.4495
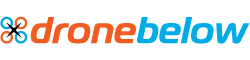