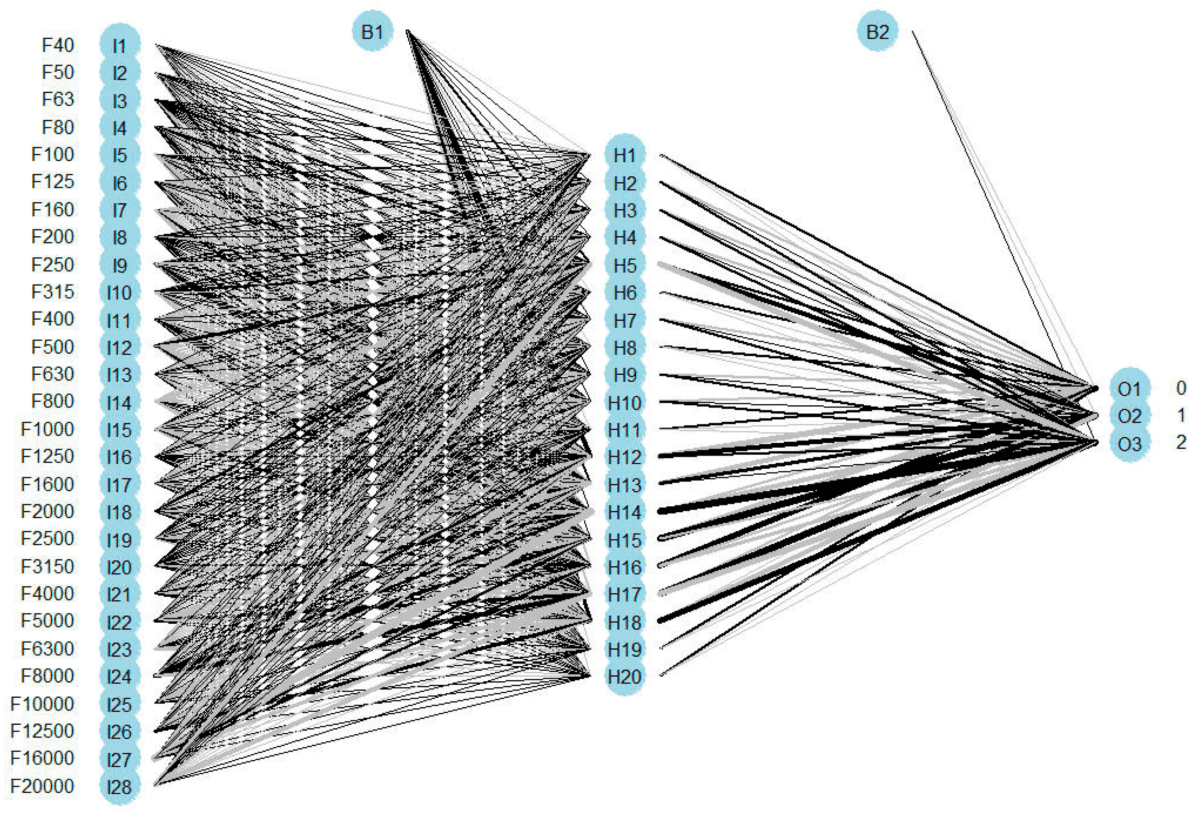
News
Fault Diagnosis of Drone Propellers Using AI
With the extensive use of unmanned aerial vehicles (UAVs) or drones maintenance problems with regard to the propellers are being studied in detail by Italian researchers.
A defect in the propellers of a drone are highly likely to cause the drone to fall from the sky which creates the obvious safety issues for people and property below. Now, researchers have tried to analyze the problem from different points of view-like supervised classification method, SVM (support vector machines); monitoring system to identify eventual faults of UAV motors and propellers.
In this new study the researchers take a unique approach, by focussing on the measurement of noise emitted by the drone and the varied noise patterns to build an artificial intelligence (AI) driven classification model to detect unbalanced blades in a UAV propeller.
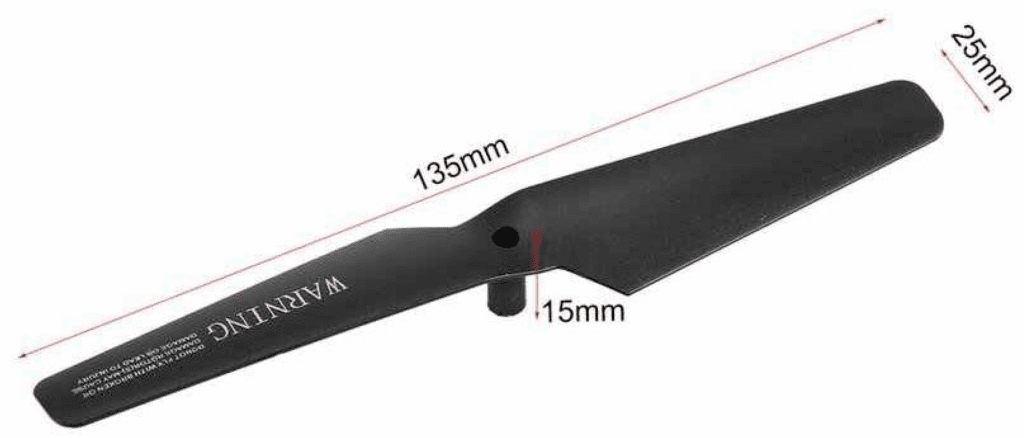
Blade shape and size.
The study aimed to develop a procedure for checking the operating conditions of a UAV. To simulate the faulty condition, two strips of paper tape were applied to the upper surface of a propeller blade. The paper tape created a substantial modification to the aerodynamics of the blade, and this modification characterized the noise produced by the blade in its rotation. Then, a model based on artificial neural network algorithms was built to detect unbalanced blades in a UAV propeller. In practice, the system can be used in the checks procedure of the operating conditions of the UAV, similar to the pre-flight checks carried out before each aeroplane flight. This methodology can be particularly advantageous in that any anomaly will be highlighted before it causes an accident.
The test is to be carried out indoors; the noise produced by the UAV is measured and eventually an unbalance in the propeller blade can be identified.
Materials and Methods
In their tests the researchers used the measurements of the noise produced by the UAV in three operating conditions: balanced blade; unbalanced blade 1 and unbalanced blade 2. These measures were processed to extract features that were later used to train and test a classifier based on an artificial neural network.
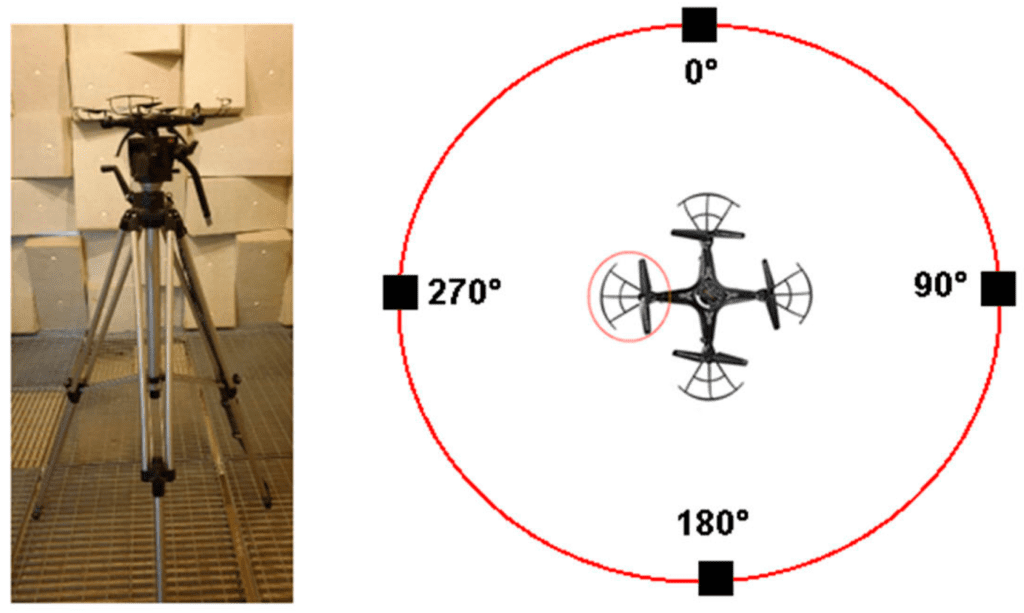
UAV anchored to the tripod and positioned in the center of the anechoic chamber (to the left) and position of the microphones (to the right) used to record the noise emitted from the UAV (1.2 m from the blade).
The data obtained from the acoustic measurements of the noise produced by a UAV were used to train a model based on neural networks in order to create a system capable of detecting an imbalance in a quadrotor’s propeller. Machine learning techniques are particularly efficient for the early recognition of operating anomalies.
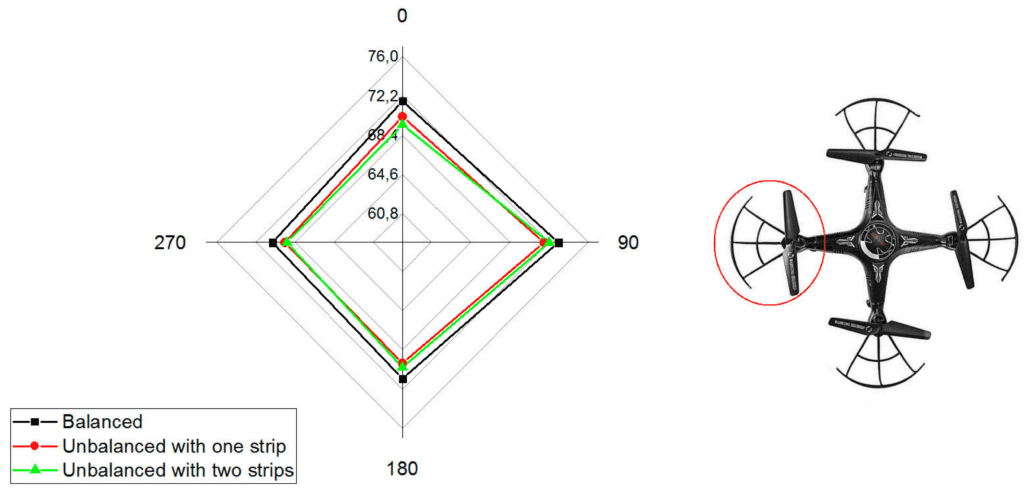
Sound pressure level (dB Lin) for the three operating conditions at the 4 microphone positions. The top view of the UAV is shown on the right with the propeller to which the unbalance was performed highlighted.
The choice of algorithm was based on the following considerations. Neural networks are widely used in pattern recognition because of their ability to generalize and to respond to unexpected inputs. In the training phase, the network learns to recognize various specific models based on the labels associated with them. In the execution phase, the network associates a specific unique label related to each model identified and if it identifies a pattern not associated with a label, then the label least different from the input is selected. In this way, the model based on neural networks shows its capacity for generalization.
The UAV under analysis is a radio-controlled quadcopter equipped with a high-quality ABS hull. The device has four feet, which provide support in landing operations. The drone is equipped with a remote control with a frequency of 2.4 GHz with a control distance of 80 m. A 6-axis gyroscope allows for strong balance in the air and precise hovering, even when there is a light wind due to the four-axis structure.
The paper tape on the propeller denotes a substantial modification of the aerodynamics of the blade, and this modification characterizes the noise produced by the blade in its rotation. It also creates a surface with a friction that is substantially different from that provided by the blade wing profile. Thus, the lift of the propeller is also modified.
For each of the three configurations- acoustic measurements were taken to characterize the UAV blade sound emissions. Measurements were carried out in an anechoic chamber (4.40 m × 4.40 m × 4.50 m), covered by absorptive fiberglass wedges. This is a laboratory environment was customized to reduce the reflection of signals on the walls as much as possible. To simulate flight conditions, the UAV was anchored to a tripod using plastic clamps and placed in the center of the anechoic chamber. The choice to position the UAV on the tripod was dictated by the need to create a fixed source-receiver system, in order to reduce the positioning error and obtain good signal acquisition. The instrumentation used for acoustic measurements included:
The sound level meter which was placed on a tripod at the same height as the UAV. The measurements were made in 4 positions placed at angles offset by 90 degrees on the circumference with a 1.2 m radius, in the centre of which the UAV was placed. Three measurement sessions were performed, corresponding to the three operating conditions of the blade (balanced, unbalanced with one strip of paper tape, and unbalanced with two strips of paper tape). For each measurement the UAV was brought to the maximum available speed and maintained at that condition for about 10s.
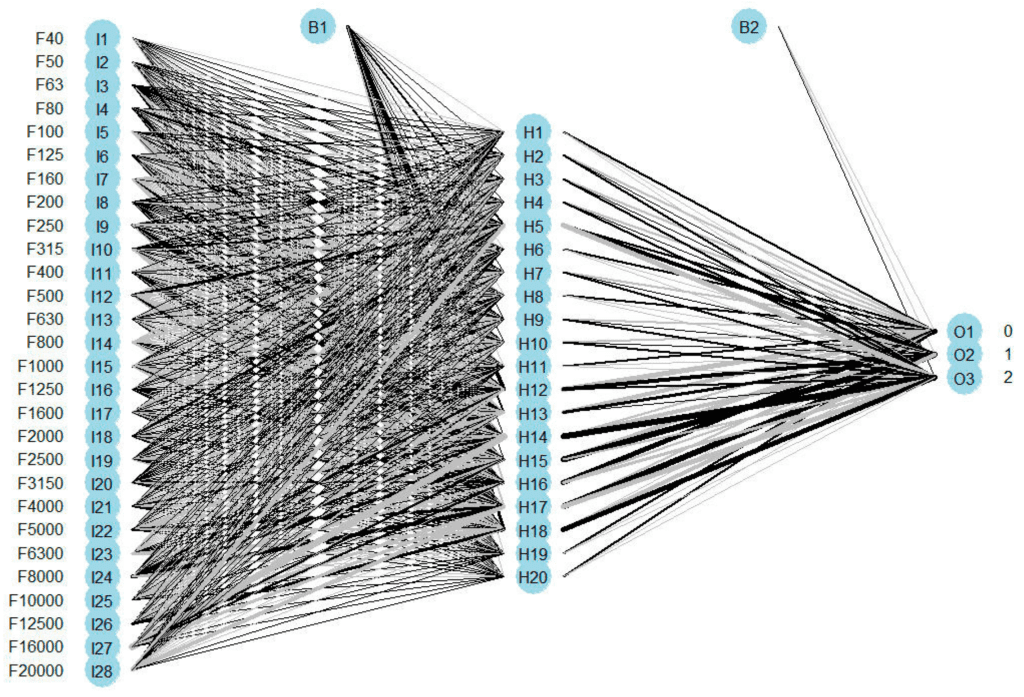
ural network architecture with weights and biases highlighted (0 = balanced, 1 = unbalanced with 1 strip, and 2 = unbalanced with 2 strips). The graph also shows the weights assumed by the connections. The color of the line indicates the contribution of the node: black indicates a positive contribution while gray indicates a negative contribution. The thickness of the line indicates the weight: the greater the thickness the greater the value assumed by the weight and the bias.
Conclusions:
An appropriate number of observations were extracted and these data were pre-processed. Subsequently, a model based on artificial neural network algorithms was built to detect unbalanced blades in a UAV propeller. This model showed a high value for accuracy (0.9763), indicating a high number of correct detections and suggests the adoption of this tool for fault diagnosis applications.
Further research will focus on the use of real damaged blades to better simulate the faulty condition. Furthermore, a possible on-board control system will be used to allow real-time fault detection during the flight. Future developments also include methods for extracting features and choosing classifiers based on other machine learning algorithms to improve performance.
The research team: Gino Iannace, Giuseppe Ciaburro and Amelia Trematerra of the Dipartimento di Architettura e Disegno Industriale, Università degli Studi della Campania Luigi Vanvitelli, Italy.
Citation: Fault Diagnosis for UAV Blades Using Artificial Neural Network, Robotics 2019, 8(3), 59; https://doi.org/10.3390/robotics8030059
Gino Iannace*, Giuseppe Ciaburro and Amelia Trematerra, Dipartimento di Architettura e Disegno Industriale, Università degli Studi della Campania Luigi Vanvitelli, 81031 Aversa, Italy, https://www.mdpi.com/2218-6581/8/3/59/htm
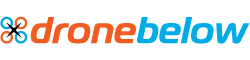