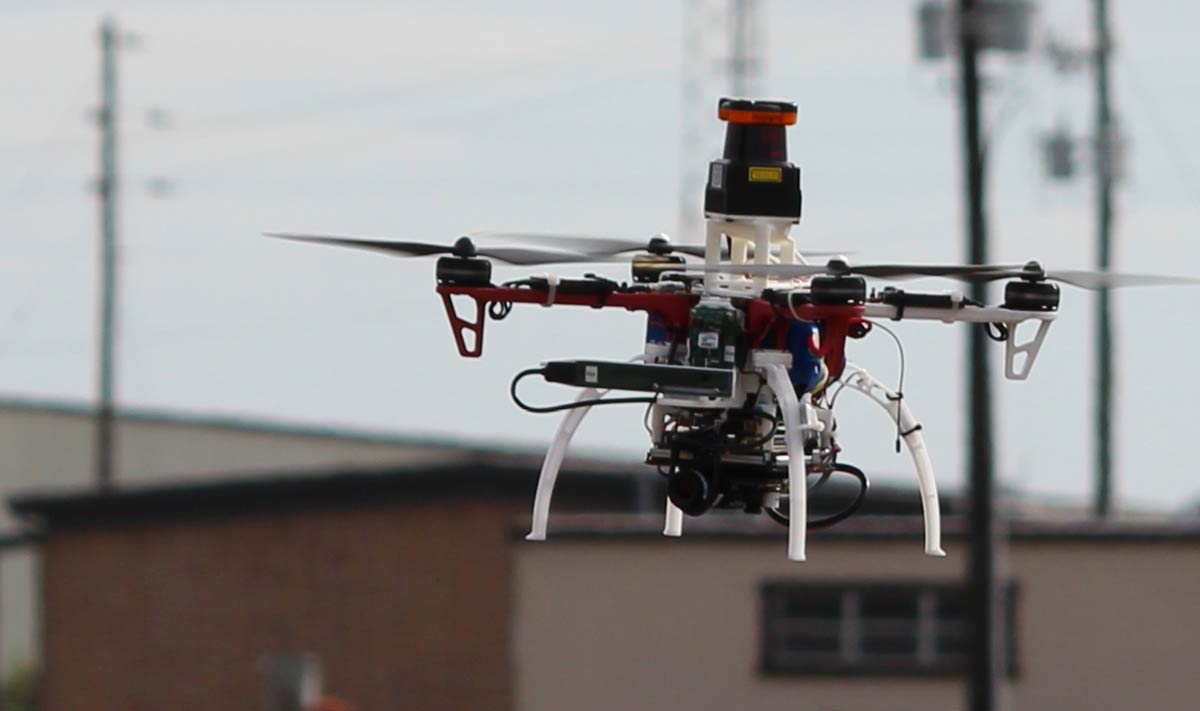
AI
MIT NanoMap Crash-Proofs Drones by Modeling Uncertainty
MIT NanoMap Crash-Proofs Drones by Modeling Uncertainty

Drone delivery is definitely a growth industry – consider the recent patent published by Amazon, or the expansion of development and testing by Drone Delivery Canada into the USA. These projects however focus largely on delivery to rural areas, with concerns such as geolocation and BVLOS taking primacy. Cities present an entirely different set of problems to solve – the ability to dodge obstacles whilst traveling at speed is complex, particularly for smaller drones that are limited in terms of flight time. The ability to avoid crashing in real time dynamic environments whilst achieving optimum speed and accuracy is what optimizes the functioning of a drone – and if done successfully is certain to benefit the growth of industries such as drone delivery.
Artificial intelligence has helped counter the challenges posed for drone navigation in real environments, and the most recent development in the field is the ‘NanoMap’ created by a team led by MIT’s Computer Science and Artificial Intelligence Laboratory.
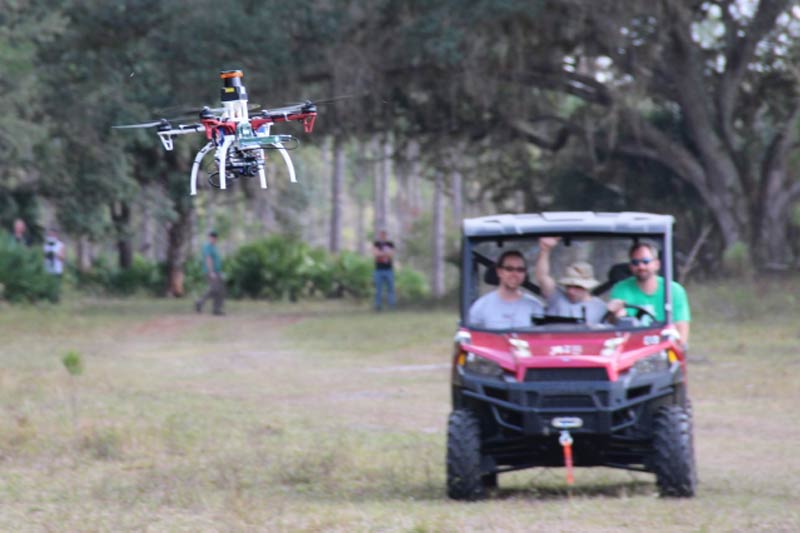
Researchers trail a drone on a test flight outdoors | Photo Credit: Jonathan How/MIT
Until now, SLAM has been the popular in building drone technology. It stands for (Simultaneous Localization and Mapping) technology which feeds information of the surroundings to the drone supported by predetermined maps and images. However, even if an object is slightly out of place, the drone might crash into it.
Several drone software incorporate GPS signals with inertial measurement units for navigation. Sensors such as cameras or lidar are used to locate obstacles, and a drone’s speed is determined by its size, the number of obstacles it faces around and the accuracy of the sensors. In a rapidly CHANGING environment like a bustling city suburb the constant change in position of objects in the drone’s path make these backend calculations and processing redundant and time consuming leading to higher probability of a crash.
The ‘NanoMap’ deals with this very issue. Instead of SLAM it uses the drone’s uncertain position to the system’s benefit, in that it, the system, uses depth-sensing to measure the aerial vehicle’s surroundings, continuously, thereby allowing it to not only plan for its next moves but for movements a few steps ahead. By forcing the UAV to constantly reconsider its position, obstacle avoidance was continuous allowing the drone to fly at a consistent 20 mph pace.
Graduate student Pete Florence, the lead author of a recent research paper based on similar ideas, stated that the approach employed here is far more reliable than traditional map-based navigation when it comes to thick, concentrated environments.
NanoMap, uses information requisite for the vehicle’s next steps only, as it needs to plan accordingly, thus reducing the processing time leading to faster reactions. It uses a depth-sensing system to compile a series of measurements about the drone’s immediate surroundings. This assists it in making motion plans for its current field of view and anticipatory plans for movement around the hidden fields of view that it has already seen, making the action crash proof.
Florence says that he has plans to integrate NanoMap with SLAM mapping to get the ensure the best results. NanoMap has been tested in a forest and a warehouse, as well as in simulated environments. Improving the accuracy of the flight is paramount rather than increasing the speed of the drone, Florence says. “We want to bring it from 99 percent reliability to 99.9 percent, and as many more nines as we can get.” His work is supported in part by DARPA’s ‘Fast Lightweight Autonomy’ program aimed at better machine navigation in cluttered environments.
How useful was this post?
Click on a star to rate it!
Average rating 5 / 5. Vote count: 1
No votes so far! Be the first to rate this post.
We are sorry that this post was not useful for you!
Let us improve this post!
Tell us how we can improve this post?
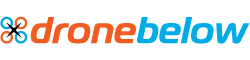