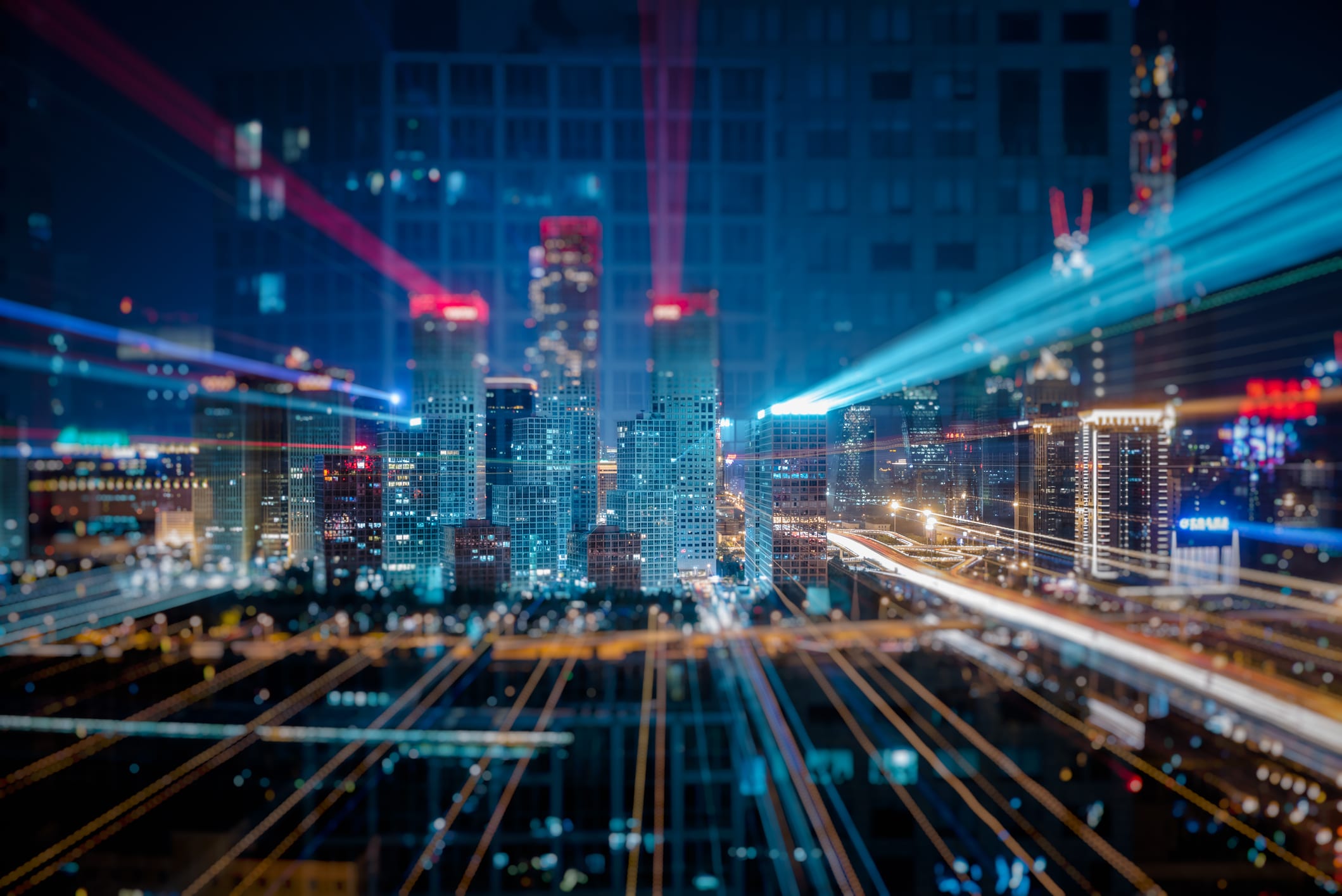
News
Improving UAV Backhaul Networks
An introduction to backhauling
In the recent years, there has been rigorous research and development aimed at effective and efficient means of backhauling data or information to a point from which it can be distributed over a network such as television broadcasts the sole reason for which being that backhaul is often the bottleneck or limiting factor of a ground network. UAV networks being developed for transfer or backhauling of data from sub-networks to the core-network are a result of the said developments to improve backhaul performance that is directly linked to network speed.
Problems associated with UAV backhaul networks
However, UAV backhaul networks have their intrinsic problems owing to different factors as discussed below:
- Network performance: The movements and locations of UAVs used for backhauling are supposed to be adjusted to ensure comfortable network performance.
- Power utilization: UAVs have limited storage capacity for batteries, which has serious impacts on the range and operating conditions of a UAV since they are both directly proportional to power consumption in a UAV. UAVs are therefore, operated at optimum conditions for efficient power consumption.
- Bandwidth allocation: The gross number of bits that can be transferred over a time interval is to be improved as much as possible. However, increasing data transfer rate or bandwidth consumes other resources such as power. The bandwidth, therefore, is maximized within constraints in terms of resources.
Considering the constraints involved with resource and bandwidth allocation, a tradeoff between the two is realized; implying that quality of network performance cannot be pursued while disregarding utilization efficiency of different resources. The operation of UAVs forming a backhaul network is supposed to be optimized for effectiveness and feasibility.
A research article published on 5th October, 2018 by engineers from the Army Engineering University of PLA at China, titled, Joint Power and Bandwidth Allocation for UAV Backhaul Networks: A Hierarchical Learning Approach, addressed the above mentioned problems associated with UAV backhaul networks.
The aim of the article is to solve the problem posed by the trade-off between resource and bandwidth utilization. The article considers both: (i) the delay performance or latency of the network, and (ii) the resource utilization efficiency critical to the networks; precisely because of the applicative constraints relevant to UAV usage.
The solution to the said problem of optimization was pursued using a Stackelberg game, which as per game theory, involves a leader and followers acting towards the achievement of the best possible solutions in an economic system. To operate or simulate this game, the Chinese engineers designed a Hierarchial Learning Algorithm (HLA) to reduce the complexity of processing in the CPU or controller as well as finding best resource allocation strategies.
Relay and Extended UAV model
Relay UAVs (R-UAVs) were used to relay data between the base station and mobile stations; the mobile stations being Extended UAVs (E-UAVs). R-UAVs and E-UAVs, combined, form the backhaul network in the form of a drone cluster, with the R-UAV being the cluster head. The E-UAVs distribute bandwidth or data evenly amongst themselves and transfer this data to R-UAVs while minimizing time wasted during this data transfer process. The R-UAV, however, can use all of the bandwidth by itself. The E-UAVs save energy by ensuring power-efficient data transfer. To use all of the bandwidth, the R-UAV is equipped with a half-duplex mechanism whereas the E-UAVs use two-phrase processes for transferring information. Such resource allocation method makes UAV backhaul network more efficient. It can realize an optimal resource allocation and delay improvement.
Stackelberg game
The resource allocation optimization and the delay or latency improvement are modeled in the form of a Stackelberg game. As already discussed, a Stackelberg game involves a leader and followers; which in this case are the R-UAV and multiple E-UAVs. The Stackelberg Equilibrium is calculated using induction. Afterwards, the leader (R-UAV) finds the optimal strategy based on the observation of the responses of followers (E-UAVs).
Hierarchical Learning Algorithm (HLA)
A hierarchical learning algorithm (HLA) is designed for the purpose of moving the drone cluster to Stackelberg Equilibrium, while using interactive machine learning at different levels during the computational process.
Results
The designed HLA was tested using a simulation to observe its effectiveness. Based on simulations that studied system latency, energy efficiency, power consumption and number of E-UAVs used, the proposed HLA was established to be an effective mode of allocation of resources as it successfully improves power consumption and reduces system delay.
Citation: Yang, T.; Yao, K.; Sun, Y.; Song, F.; Yang, Y.; Zhang, Y. Joint Power and Bandwidth Allocation for UAV Backhaul Networks: A Hierarchical Learning Approach. Preprints 2018, 2018100100 (doi: 10.20944/preprints201810.0100.v1). https://www.preprints.org/manuscript/201810.0100/v1
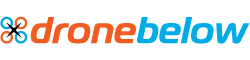