AI
Ericsson Looks to Identify Uncertified Drones with AI and Machine Learning
An Ericsson white paper reports an interesting study on drones and networks with in-depth look at the use of machine learning to separate drones from ground devices.
In their paper “Drones and networks: Ensuring safe and secure operations“, researchers Dr. Xingqin Lin, Henrik Rydén and Sakib Bin Redhwan describe how mobile networks are well suited to support low-altitude drone communication and to be integrated with drone traffic management systems to enhance the safety and security of drone operations.
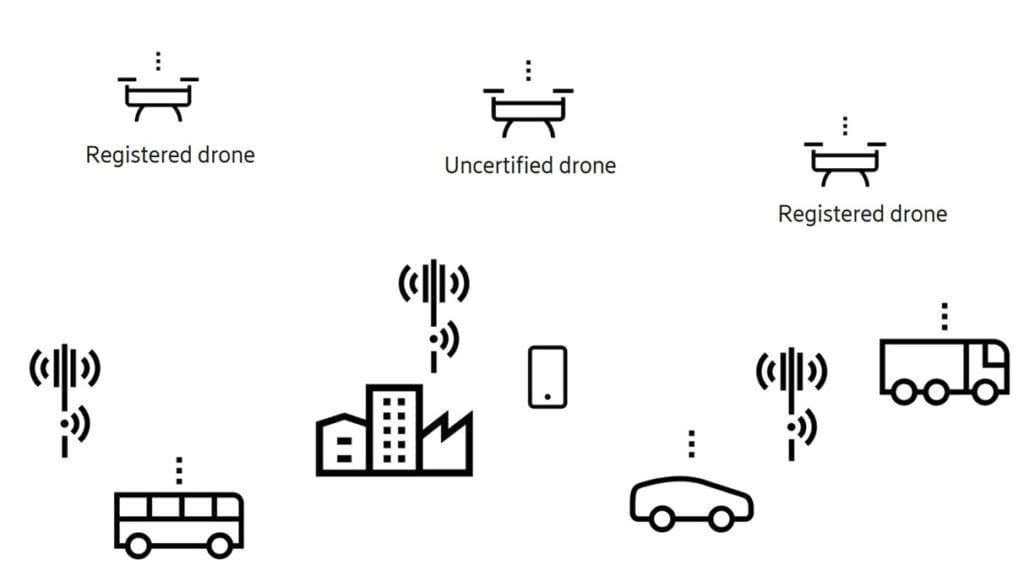
Authorized and unauthorized drones co-existing in a mobile network
Many use cases require drones to transmit video feeds to their flight controllers, imposing heavy uplink traffic load on the networks. Therefore, it is important that mobile networks identify if a user equipment (UE) is a drone UE or a regular ground UE, in order to provide the right service optimization for drone UEs while ensuring the performance of ground UEs is not impacted.
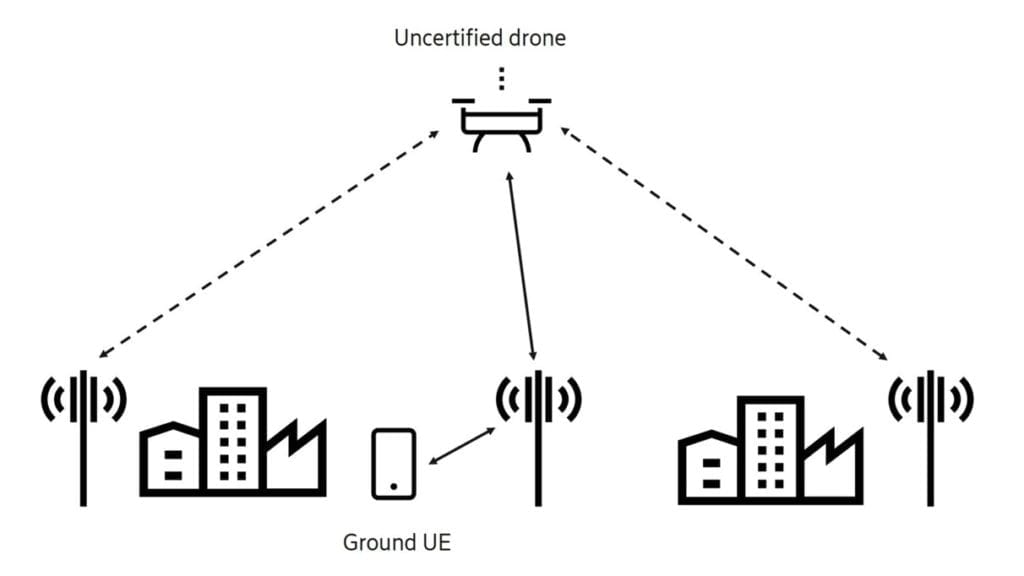
Radio link characteristics for aerial and ground-based devices
Aerial radio channels have a higher likelihood of line-of-sight propagation due to the absence of obstacles, so an uplink signal transmitted from a drone UE may reach multiple neighboring non-serving base stations and thus cause increased uplink interference.
As soon as an uncertified drone is detected, interference mitigation techniques can then be initiated.
Machine learning, the science of programming machines enabling the machines to learn from data, can be utilized for this purpose- the logic being:
The radio link characteristics and mobility patterns are different for devices in the sky and devices on the ground. A drone UE operating at a high altitude is expected to have a close to line-of-sight propagation environment that leads to low variance of Reference Signals Received Powers (RSRP) of the strongest cells. Similarly, the Received Signal Strength Indicator (RSSI) statistics of drone devices are different from those of regular ground devices because a mobile device mounted on a drone may receive signals from multiple cells with similar strengths. For indoor UEs in high-rise buildings, the penetration loss of the signal entering the building will heavily degrade the signal quality in comparison to an aerial UE at similar height, enabling the classifier to differentiate the two UE types.
Different radio characteristics for ground UEs and drone UEs have been validated in field trials. Some results from the field measurements indicate that, the signal strength variation in the sky is much smaller than on the ground.
The machine learning task is then to predict UE type (drone UE or ground UE) based on the radio measurement reports sent by the UEs to the network.
One option could be a central entity stores all the data and trains a single machine learning model. Such an entity could be a network node or a device in the cloud that may be further integrated to be part of a UTM system. Alternatively, machine learning models can be trained per cell or group of cells. So, each cell or group of cells can run a model locally and exchange the output with neighbors to collaboratively carry out the uncertified drone detection task.
Dr. Xingqin Lin is a Senior Researcher and Standards Delegate with Ericsson Research Silicon Valley. Henrik Rydén is an Experienced Researcher in Radio at Ericsson Research in Kista, Sweden. Sakib Bin Redhwan is a Researcher in Network Architecture and Protocols at Ericsson Research in Linköping, Sweden have presented their findings in this paper.
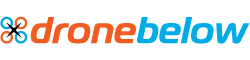