Tracking Pedestrians with Drones
The use of small/miniature unmanned aerial vehicles (SUAV) or miniature drones for a variety of applications has been on the rise. SUAVs are very useful for visual detection and tracking due to their efficiency in capturing scenes. This feature of drones is now being put to use in the detection and tracking of moving pedestrians.
Researchers Seokwon Yeom and In-Jun Cho from the School of Computer and Communication Eng., Daegu University in Korea have been working on deploying drones to track pedestrian movement in order to help improve traffic management.
The SUAV is cost-effective for capturing aerial scenes. The camera can be easily built and manipulated in order to capture the scene of interest in a long distance, however, the computational resources of the drone are often limited to processing high-resolution video sequences in real time.
In their paper this research team addresses the detection and tracking of multiple moving pedestrians by an SUAV or drone. In their experiments, researchers used a total of 13 moving pedestrians and one car is captured at a height of 15 m by a drone. Visual detection is performed through frame subtraction, with thresholding and dilation operation, and false alarm removal. Each frame is subtracted from a past frame, separated by a constant interval. Then, thresholding generates a binary image and dilation is applied to the binary image to produce candidate target regions. Finally, false target regions are removed, with the known size of the real object. The centroids of final region of interest (ROI) windows are considered x and y positions, which are fed to the next tracking stage as measurements. This detection approach neither requires intense training process nor heavy computational burdens. Therefore, this method is suitable for autonomous stand-alone aerial video surveillance systems with a drone, which have limited computational resources.
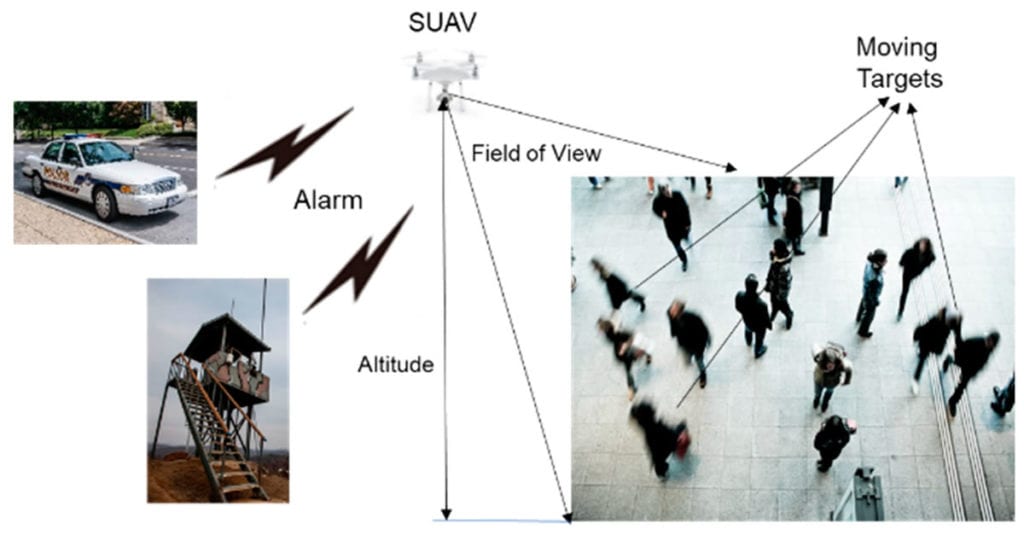
Illustration of aerial surveillance with a small unmanned aerial vehicle (SUAV)
For state estimation, the IMM filter estimates the state of the target and the covariance matrix. Nearly constant velocity (NCV) models with two different covariance matrices of the process noise are assumed for the dynamic states of the target. For data association, a gating process excludes measurements outside the validation region of each target. The nearest measurement-to-track association scheme assigns one measurement to the closest track based on the statistical distance of the residual. This nearest neighbour (NN) approach is efficient for the visual tracker because the false measurements cannot appear in the area of a target of interest.
The major contributions of this paper lie in the following:
(1) Integration of visual detection based on image processing and a target tracking derived from statistical estimation. It was noted instant dynamic state estimates, such as position, velocity, and acceleration can be made available using the proposed method.
(2) No massive training data is required for target detection and tracking. Thus, this method can speed up the process, with less computational resources. Drones have limited computing power, memory, bandwidth, and battery; thus, a small computational load is required for a drone system;
(3) A practical solution is proposed for autonomous stand-alone aerial surveillance.
The SUAV can move to any location where CCTV cameras cannot be installed and hover or maintain its position. It is very low cost and can be operated by non-experts. Thus, it is useful for combat missions, counter terrorist operations, or search and rescue in military or commercial use. The SUAV continuously monitors human movement within the field of view of the attached camera at a certain altitude. If any threat is detected, an alert is sent to the authorities.
Experimental Set-Up
A drone (DJI Phantom 4 Advanced) was used to capture moving objects. The drone, with an attached gimbal and camera which can tilt the camera within a 120° range (−90° to 30°). The camera pitch was set to −30° during the experiments.
The drone ascended to a height of 15 m and stayed still as a stationary sensor (platform). It maintained its position while capturing video sequences of moving objects in the campus area. In their paper the researchers document their findings through various pictures captured by the drone camera from several different heights and angles.
For example- at a height of 100 m, to better visualize nearby buildings and structures. Another picture is a sample frame extracted from the video.
Conclusions
In this paper, several moving people and cars were captured by an SUAV. The objects were detected based on frame subtraction. Ten targets were tracked with the Kalman and IMM filters. Experimental results show that moving objects were well detected and tracked with good accuracy. The number of filter modes and the target dynamics of each mode, such as the process noise variance, should be determined properly to cope with the manoeuvring of multiple targets. —the average detection rate is 96.5%. When the two-mode IMM filter is used, the minimum average position and velocity RMSE obtained are around 0.8 m and 0.59 m/s, respectively.
For security and defence applications, the trajectories and the states of targets can be transferred to a control tower in real-time. Also, this system is suitable for people counting in a crowd area. Fully autonomous and stand-alone aerial video surveillance systems are very useful in commercial as well as military/government applications. In this work, the drone was fixed in the air as a stationary sensor (platform). Target tracking with a moving platform remains a subject for future study.
Citation: Detection and Tracking of Moving Pedestrians with a Small Unmanned Aerial Vehicle, Seokwon Yeom and In-Jun Cho, Appl. Sci. 2019, 9(16), 3359; DOI: 10.3390/app9163359, https://www.mdpi.com/2076-3417/9/16/3359
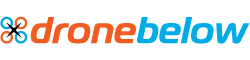